Now that the dust has settled on the interest in generative AI caused by the launch of ChatGPT, owners and operators of commercial real estate are taking a sober look at how AI and machine learning can deliver value for their business.
But before we dive into the details…
A quick primer on AI and machine learning
Artificial Intelligence (AI) can be described as machines that can duplicate or exceed the cognitive functions of humans.
AI has been around for a long time, but it is only recently that models have been able to generate outputs without any human input other than data.
Machine learning (ML), on the other hand, is a subset of AI that enables machines to learn from data and improve automatically when trained by human intervention.
Machine Learning is used to identify patterns and relationships among data sets, recognize anomalies, and make predictions based on historical data.
Both of these strategies promise to help identify and leverage insights that would otherwise take years to glean by manual analyses.
Practical Applications of New AI Capabilities in Commercial Real Estate
The applications and benefits of AI and ML in CRE building operations are vast, but the following are a few practical examples:
1. Triple Net Tenant Energy Insights
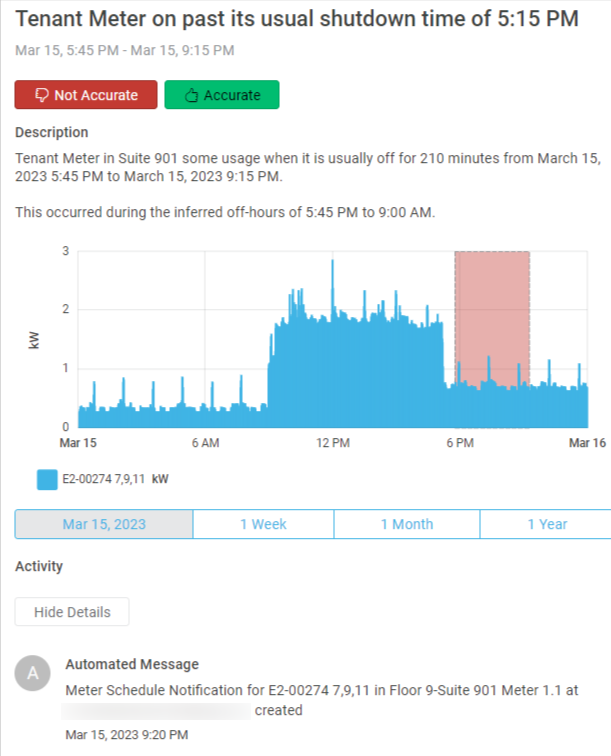
Triple net landlords (especially those that are publicly traded) have been deploying shadow metering to capture tenant-controlled utility data for reporting purposes.
As a byproduct, there is real-time transparency into tenant consumption for the first time. Because this is meter data (rather than tied to specific pieces of equipment), deriving insights are more difficult. AI is allowing technology providers to disaggregate this data and provide efficiency insights to tenants.
While not a direct benefit to the landlord, providing this type of value add service is being prized as more and more tenants take sustainability into account in their leasing decisions.
2. Predictive Maintenance
What has been called predictive maintenance in the past has often been fault detection in reality.
The difference is that fault detection is rule based. For example, if power draw on a supply fan falls below a certain threshold, the system can be confident that the belt has snapped.
Predictive maintenance on the other hand requires a much more sophistication. There is no rule to follow. Instead, AI can monitor a piece of equipment relative to thousands of other pieces of equipment and identify patterns that indicate degradation immediately preceding a fault.
That means that less time can be spent on unnecessary maintenance, and that big issues are caught before a breakdown occurs, saving significant costs (the average repair is 10X the cost of what preventative maintenance would have been).
3. Vacancy Insights:
When a property that recovers utility costs from tenants (often via submetering) has turnover, vacant units still tend to consume energy.
AI can not only make the connection between occupied spaces and energy consumption, but target which specific loads can be turned off or modulated to capture the energy savings potential. This is perhaps the most overlooked piece of the decarbonization puzzle.
4. Work Order Generation:
Everyone wants their issues to be resolved in a timely manner, but no one (tenants, property managers, engineers, etc.) like writing tickets. When tickets are sparse on details, it requires additional trips by operators or maintenance vendors, unnecessarily driving up costs.
Now, AI is getting smart enough to predict and write out work orders for the user depending on the location or equipment in question, timing and other factors.
5. Bidding and Vendor Management
Too many decisions are defaulted to the vendors with whom there’s an existing relationship. This complacency leads to higher costs and worse service.
Now, when equipment breaks down and a repair is necessary, AI can automatically generate an RFP, solicit bids and provide a short list to be reviewed.
Conclusion
While the practical application of machine learning has already generated value in commercial real estate, the potential for AI is vast.
As AI algorithms become more sophisticated, and more data is captured, building operations will evolve from reaction to prediction, engaging owners, operators and tenants in novel ways.
The key to remember is that an enormous quantity of data is required for these algorithms to work. While quality will always be important, quality assurance is generally easier when related to maintenance, utilities and capital projects, than to things such as leasing and ownership (where the data is more qualitative).
At nearly 30 billion hours of data, Enertiv has one of, if not the largest dataset in the industry. Schedule a demo to see how this can translate to value for your portfolio.