Introduction
Recent advances in fault detection and diagnostics software promise to deliver something that has never existed in commercial real estate: true transparency into the health of building systems and the indoor environment, regardless of building type or size.
In many commercial real estate portfolios, fault detection requires making judgment calls based on incomplete data. In a good scenario, this data is derived from sensors connected to a robust Building Management System (BMS). In the 90% of buildings that do not have a BMS installed, the only datasets available are pieced together from spreadsheets, maintenance logs, and utility bills.
Now meters and sensors connected to the cloud can capture real-time data from hundreds of pieces of equipment per building for a fraction of the cost of a traditional BMS. These data sets can be analyzed and synthesized into specific insights about performance. For a sense of scale of the amount of data: a meter that takes readings of equipment performance every second will have generated 86,400 data points in a single day. Multiply that by hundreds of pieces of equipment per building and operators can now utilize tens of millions of new data points per day.

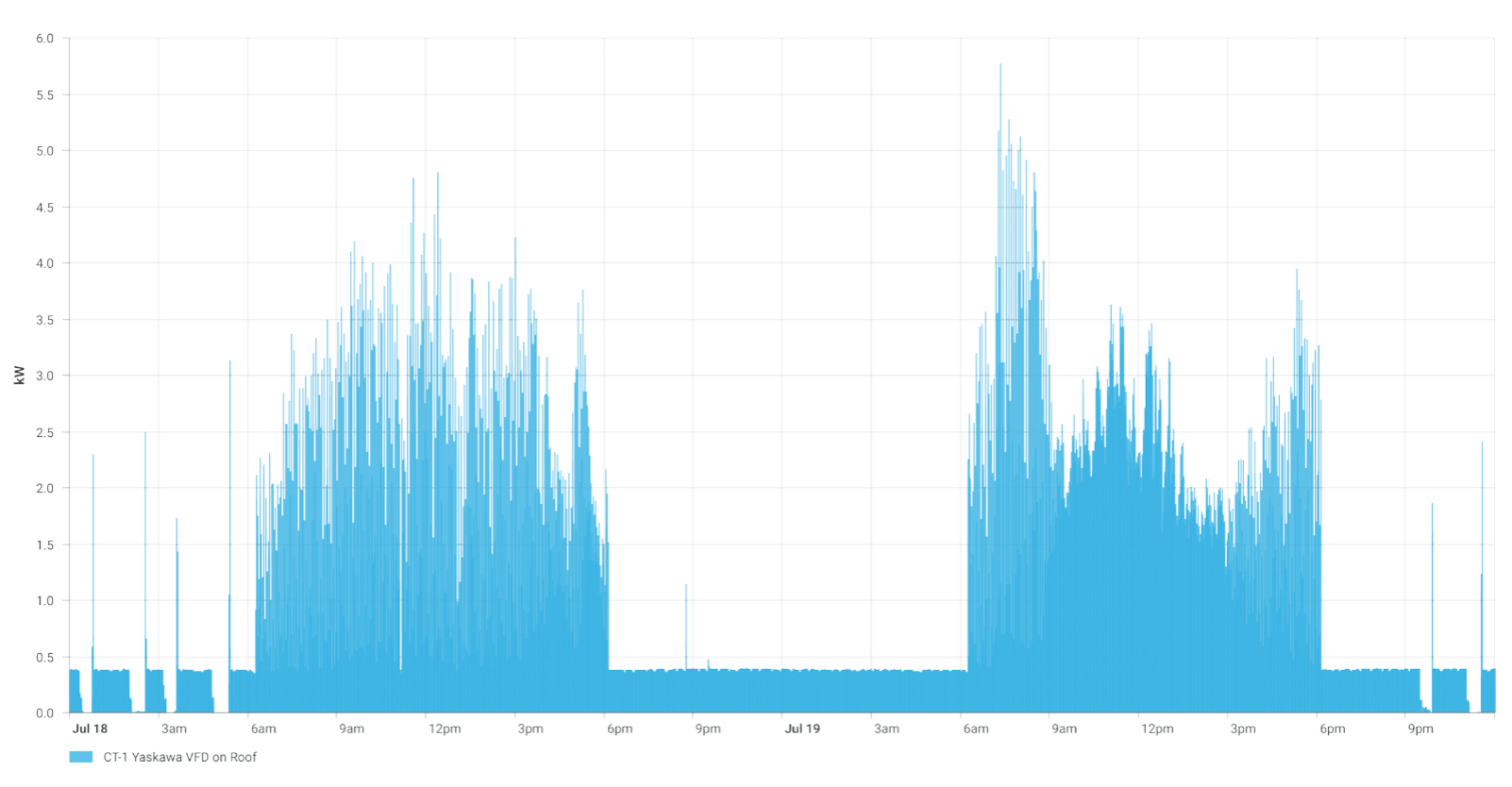
But millions of data points are worse than useless to building operators. Raw data is time consuming, confusing, and not actionable. That is where the advances in fault detection and diagnostics come into play. Enormous data sets, combined with novel analytical techniques such as machine learning, are enabling software programs to identify issues far beyond the what building operators and engineers are familiar with.
For example, many traditional fault detection and diagnostics solutions operate based on thresholds. Some equipment is designed to run at all times, and so an alert that a system is no longer drawing any power can drastically shorten maintenance resolution times by directing operators to the right piece of equipment in real time. The logic here is simple: If power drops below a defined number, then trigger an alert.
On the other hand, equally damaging issues cannot be identified with such simple logic. One example is equipment short cycling, which is when equipment shuts down and starts up in rapid succession. This can be very detrimental to equipment life and wastes energy-related costs. As the length of each cycle can vary wildly, there is no simple logic for identifying this issue; it requires a much more sophisticated analysis.
In addition to advances in the analytical capabilities of fault detection software in the cloud, the hardware deployed to collect data is becoming able to perform analyses on-site through a concept known as edge computing. Edge computing disperses the analysis of the mountain of data created by meters and sensors to the individual site of collection, instead of a requiring a centralized analysis in the cloud. This enables faster, more granular analyses, and reduces the costs involved in transferring, storing, and processing data in the cloud.
The implications of edge computing are enormous. Not only will it bring down the costs of implementing fault detection solutions, it will create the conditions for highly customized analysis. Every building is operated a little differently; instead of trying to create rules in a centralized manner that apply to a wide swath of building systems, edge computing enables software developers to “train” a device and allow it to “learn” the specifics of its environment.
This learning can go beyond detecting an issue after it occurs. With large, historic data sets, fault detection can move towards predictive maintenance, advising operators when data suggests that equipment is on the verge of failure.
In addition, the savings in data transfer and storage can translate into more useful aggregation and unit conversions. This may seem trivial, but most on-site operators do not have any context for the units of measurement that meters and sensors are capturing. For example, equipment performance is usually measured with the power demand unit kilowatts (kW), but very few operators could tell you what the baseline demand of any piece of equipment is.
Understanding this, algorithms have been deployed to convert power consumption data into units that make sense for the end user. While kilowatt hours might not mean anything to an operator, knowing the runtime hours of a piece of equipment is a very valuable piece of information that can inform maintenance schedules, startup times, and set point adjustments.
These advancements have consequences for buildings of all sizes and types, regardless of whether there is currently a central building management system installed. Fault detection does not only cover equipment which has failed or is performing poorly. Equipment that is operating perfectly, but during the wrong times are a major source of energy waste in buildings. This includes buildings with a BMS installed, where the Department of Energy estimates that improperly configured BMS results in 20% higher than necessary energy usage.
Fault detection software can infer an occupancy schedule based on actual usage data and generate an alert as soon as it detects a piece of equipment running off schedule. For Class A properties that have assumed their automation and controls means efficient operations, perhaps it is time to take another look at fault detection software to compliment existing systems.